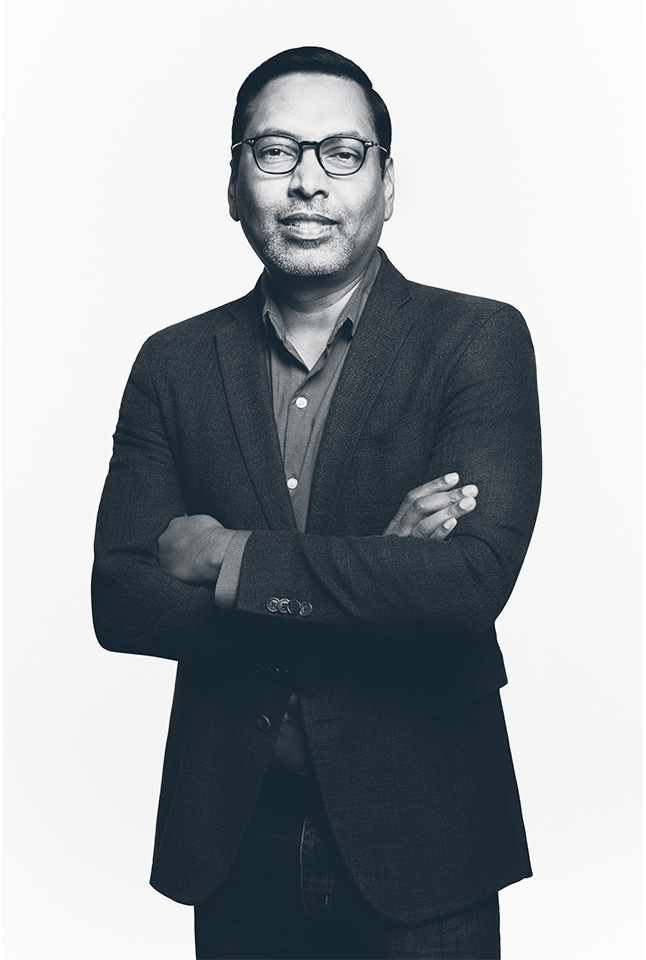
CxOs are realizing every executive in the organization is a data leader in the age of digital transformation. Whether their background is in data analytics or not, successful CxOs are navigating this transition by actively engaging with data departments to fill gaps in knowledge. They proactively build a set of practices and habits that drive useful insights. In doing so, leaders have pivoted from a defensive to an offensive data strategy and culture.
Analytics teams previously collected data, analyzed it and offered insights directly to leadership. Instead, modern data teams are much less siloed. They work with, and in support of, multiple executive officers and stakeholders in different departments, in a decentralized way, throughout the company’s digital transformation.
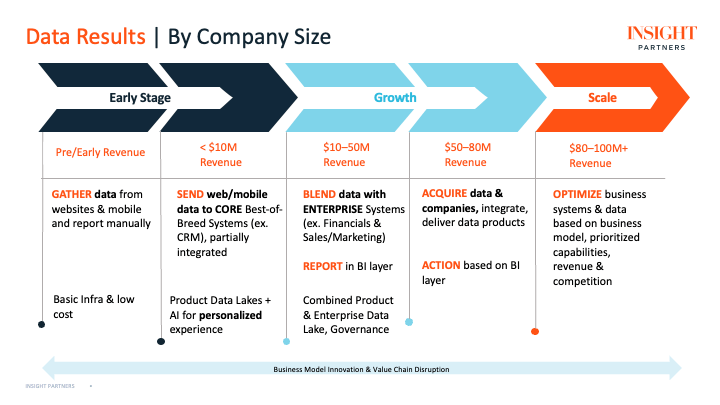
How the leadership navigates this spectrum varies from company to company. However, highly effective data leaders have established a series of best practices to guide them through the growth curve, and continue to follow these practices until they become habits. As Pulitzer Prize–winning writer and productivity expert Charles Duhigg wrote in The Power of Habit: Why We Do What We Do in Life and Business, “there’s nothing you can’t do if you get the habits right.”
1. Shift the culture and strategy
The role of data executives can be transformative. Their ability to collect and analyze data, then create real operational value from the business insights that data reveals, makes them a transformational force. It’s in any executive’s interest, therefore, to embrace data technologies. Every department stands to gain, from finance (maximizing revenues and minimizing costs) through enhancing sales and marketing practices (buyer intent, lead generation and opportunity conversion to order), to the C-suite (organizational transformation, long-term growth, customer churn and competitive strength).
Data leaders rely on data to actively predict customer behavior, and refine their own engagement practices and pipelines. How sophisticated they are often depends on where the organization is in its digital journey.
Data-driven organizations are maturing as they move beyond relying on low-level automation tools such as chatbots, to measure successful customer experiences, using this insight to predict future customer behavior. A 2021 Gartner report confirmed that customer service departments get most value from technologies that analyze customer data.
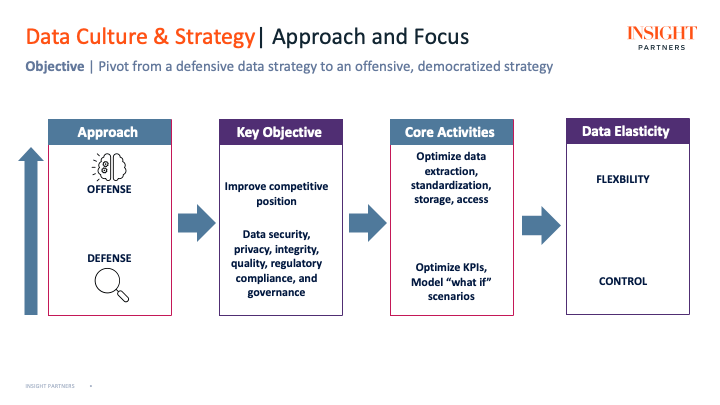
Data Culture & Strategy | Approach and Focus
2. Refine the business context
The business context in which data is collected is changing too. Increasingly, organizations are focusing on customer-centric research to enrich their analytics strategies. A large part of data leadership has focused on identifying short-, medium- and long-term metrics to better understand and predict customer behavior. Although some customer trends stay the same, others fluctuate, year by year, as the ways customers interact with technology slowly evolve.
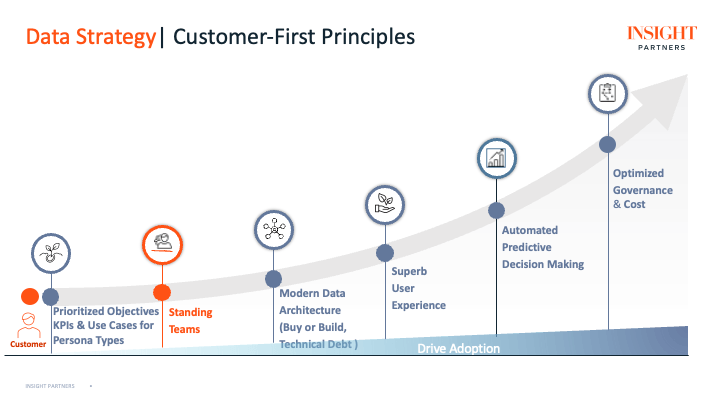
In response, business leaders are learning to modify their organization’s operations, sales and analytics strategies and to adopt more customer-focused data solutions. Data pipeline company Rudderstack provides a data platform to help organizations implement this data-driven strategy for improved customer support. The platform allows enterprises not only to track customer data, but to directly engage with the customer.
3. Grow the data pipeline with the company
As mentioned above, data strategies shift as companies grow. Early-stage companies often have a very different set of tactics — and see very different results — than multi-million dollar scaleups. As they scale, though, data leaders realize that the range and level of data-driven insight must scale with them.
Leaders often progress from planning a data strategy that merely harvests data, to one that focuses on action by using data to shape organizational decision-making at every level. This is a crucial transition, as it requires data departments to undergo a fundamental intellectual shift, from being passive collectors of data to active advisors. In this way, data streams and enterprise systems are integrated so that predictive data can actively inform decisions.
Data analytics innovator Kubit, for instance, provides a self-service behavioral analytics platform. It harnesses behavioral insight that allows organizations to optimize sales and marketing conversion.
4. De-silo the architecture
The shift towards more proactive data strategies also involves rethinking the data architecture. Previously, organizations might have organized their data by team or purpose. Now that companies need greater volumes of better quality data, siloed datasets can hinder proper access to the data.
Many data leaders are migrating from a data warehouse-centric architecture to a data lake-centric one. Data lakes provide much greater reporting capability and analytical flexibility thanks to their accommodation of unstructured data. Conversely, data warehouses require structured data while data lakes can accept almost anything: Structured data, unstructured data, media and more.
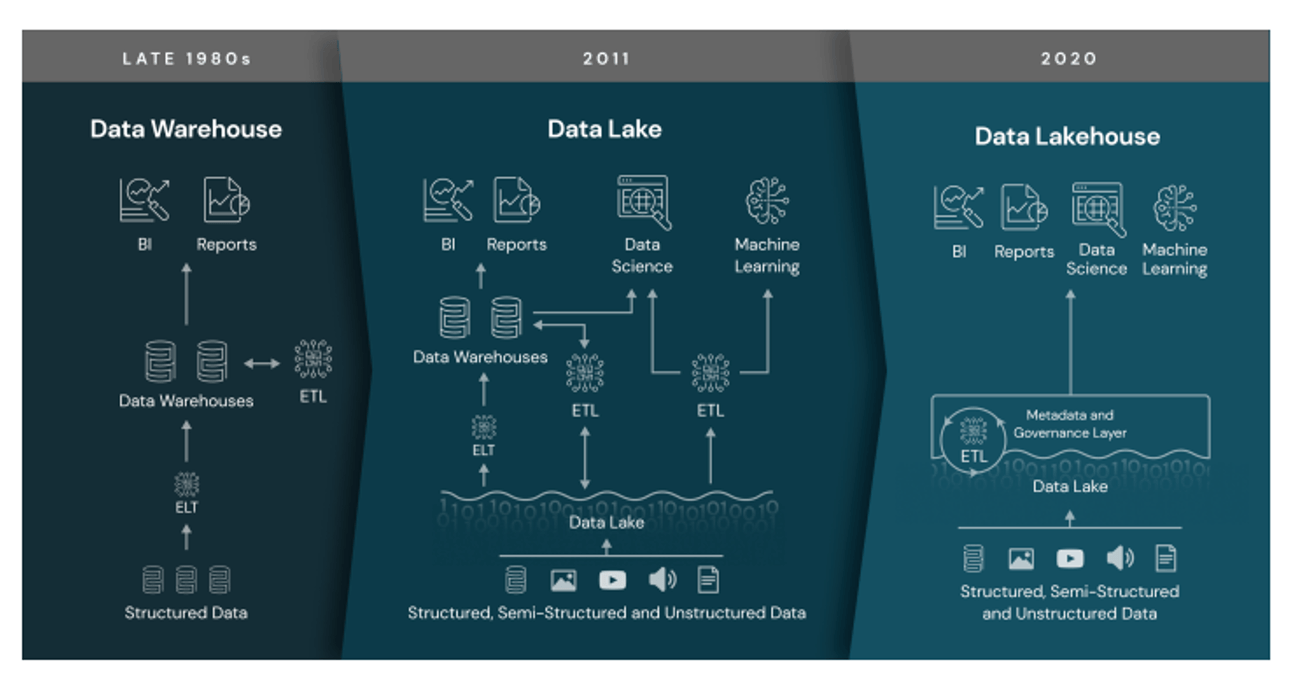
Data Architecture Evolution | Source: Databricks
However, the structured data of a warehouse works well for analytics, but is too rigid for advanced AI or machine learning (ML) models to work with. Data lakes are not without their challenges either: They provide great flexibility for AI-driven processes but are unsuitable for reporting dashboards. As a way to overcome the gaps in functionality for both alternatives, the data engineering company Databricks offers data lakehouses for both advanced reporting and agile AI.
Regardless of the implementation, executives are leading the move to a more unified, de-siloed architecture where data from multiple streams and teams can be integrated to inform better decision-making. A new trend is to master customers in the data lake versus the CRM systems, allowing for a complete 360 view of the customer available for real-time AI.
5. Build trust in data
Earlier, we described the growth trajectory of early-stage startups as they scale, in terms of their shift in focus in data strategy. This doesn’t mean, however, that DataOps principles such as governance and security take a back seat. Privacy, compliance and security remain critical to maintaining customer trust in any organization. Data leaders are always sensitive to these concerns and are constantly working to enhance trust in their company’s data policies.
Proper governance is a huge pain point for startups — Gartner’s 2021 outlook predicted that, through 2025, 80% of organizations will see efforts to scale their business fail because of a lack of modern data governance. To make matters worse, some businesses don’t even have visibility into how effective their governance policies are. Over 40% of executives surveyed had no metrics to measure whether their data governance policies were actually working.
Fixing this problem is an ongoing challenge as executives struggle with perception as much as reality. Every time a data breach becomes public knowledge, company leaders confront a tide of general suspicion and distrust from customers, even if the breach happened to a different company.
Credit bureau Experian, for instance, saw its brand tarnished by the infamous Equifax breach that exposed the financial data of 143 million Americans.
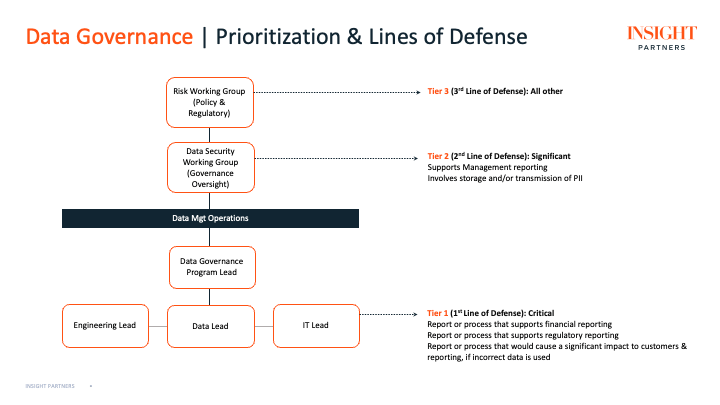
Data Governance | Prioritization & Lines of Defense
To deal with much bigger threat vectors, executives have begun instituting strict policies at every organizational level: From the adoption of tools like multi-factor authentication (MFA), to precise cloud-access control policies and updated engineering best practices for sensitive data.
Many companies prefer to buy — rather than build — their governance and security infrastructure. Rather than rolling their own authentication and security suites, enterprises opt for third-party offerings like Privacera. Privacera provides a unified suite of access control, data governance and security solutions for data warehouses. This type of third-party, all-in-one solution reduces the workload of a company’s IT and engineering teams by removing the need to maintain and constantly update security code. It also leaves security in the hands of the experts, promoting greater reliability.
6. Focus on people
Building a scalable data department in today’s dynamic, post-pandemic workplaces requires leaders to have an innate ability to collaborate with stakeholders from across the enterprise. Data teams are more decentralized, as previously mentioned, with data scientists embedded within teams from other departments such as engineering, product sales or marketing and finance.
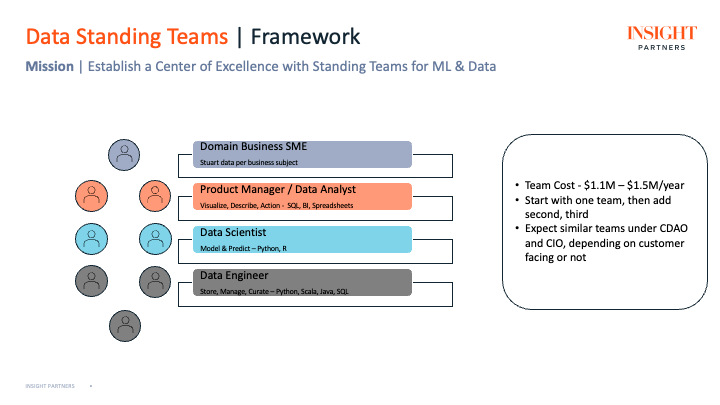
Data Standing Teams | Framework
Just as the remit of the data department has expanded, so has the role of its leaders. Previously siloed chief data officers are now chief data and analytics officers (CDAO), forming skilled teams in an environment where data-driven insight is viewed as a key competitive advantage. And for both growing and large businesses practicing new habits, “Small wins are a steady application of a small advantage,” Duhigg wrote. This collaborative mindset often becomes a culture through the entire department, enabling data teams to work more smoothly with other stakeholders.
7. Drive value
The final responsibility modern data leaders carry is the job of driving consistent and tangible value for an organization. In bringing modern analysis techniques to company teams, data executives have an opportunity to influence genuine change. Evidence shows that by being responsive to customer behavior companies can improve satisfaction, loyalty and drive new revenues.
It’s often the case that one successful project will breed curiosity in other parts of the business. Where successful proofs of concept are adopted by other departments, overall efficiencies are amplified. Data-driven optimization initiatives that help companies transition from insight to action have led to real gains. Driving this kind of optimization helps establish the importance of the rise of data leaders and CDAOs as an engine room for company credibility and growth.
New data habits enable successful digital transformation
The emergence of every CxO as a data executive has cemented the importance of data to a company’s digital transformation. As analysis methodologies become more sophisticated, and the use of data continues to evolve, the ability of leaders to form new habits will be crucial. Storytelling using data and visuals can change a company’s trajectory from being a follower to becoming a leader — as well as a disruptor — in an industry segment.
Note: Insight is an investor in Rudderstack, Kubit, Databricks and Privacera