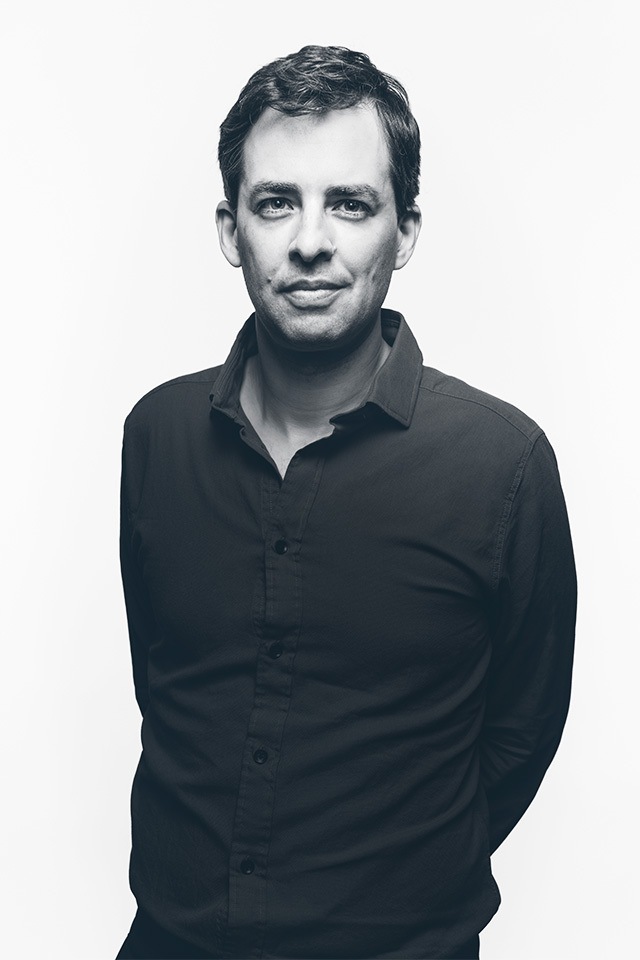
Four Key AI Challenges and How the Talent Shortage Impacts Them All
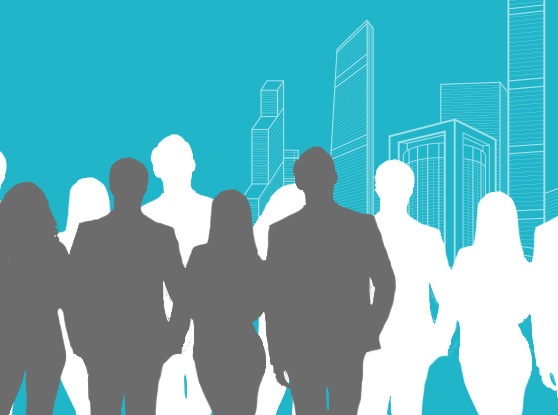
When COVID first hit, many fast-growing software ScaleUps braced for significant declines in top-line revenue, preparing to tighten their belts by reducing expenses and conserving cash in order to make it through the crisis. But many ended up being extremely resilient: They hired rapidly and even experienced accelerating growth throughout the pandemic. From May to August of 2020, during the peak period of COVID-19 closures, there were an average of 3,500 open roles across Insight Partners’ portfolio companies. ScaleUps are where a lot of the job growth happens.
As a result, one of the most significant challenges faced by ScaleUps right now is the shortage of talent – it comes up in almost every board conversation today.
This talent shortage is particularly acute for AI ScaleUps: high-growth companies using machine learning, traditional software, advanced hardware and robotics, and new communication technologies to build learning systems that can automate nonroutine tasks.
Because these companies need leaders with specialized skills across the various domains important for building and scaling AI systems, it’s even harder for them to recruit and retain workers. This challenge was explored at Insight’s recent ScaleUp:AI conference, including in my own keynote.
Here is a breakdown of a few of the technical challenges that were covered during the ScaleUp:AI event, how those challenges translate to hiring needs, and some software capabilites that are emerging to address these skills gaps:
MLOps
Data collection, preparation, and deployment are critical to a successful AI system, but they remain highly manual processes. Meanwhile, techniques like self-supervised learning, generative adversarial networks (GANs), and active learning require even more specialized skills.
Machine learning operations (MLOPs) tools can help manage and improve those processes from start to finish.
The MLOps stack has been evolving, so far, as a different tech stack from traditional analytics. There’s even a growing divergence between structured and unstructured MLOps stacks.
Lots of MLOps infrastructure ScaleUps are working to fill this skill gap with software capabilities (e.g. W&B, Run:AI, Deci AI, Rasgo, Explorium, Landing AI, etc.) but the tech frontier is continuously evolving.
Explainability and Causality
Many AI systems lack a way to trace or explain how they make specific predictions. This makes it hard to test these systems and also makes it difficult for these systems to engender trust and meet regulatory requirements.
Understanding causality improves predictions, and humans can often see causality (not always accurately) that a machine learning system will miss. Humans have a broader world model and can imagine counterfactual scenarios to simulate cause and effect relationships.
Expertise is needed to teach these broad human intuitions to the more narrow scope of today’s AI systems. New ScaleUps have emerged to manage the process of creating explainable and responsible AI, such as Fiddler and Zest AI.
Privacy and Security
Without sufficient protections, AI applications may be limited by concerns around personal data collection and use.
New techniques are emerging, such as federated multi-party computation, but these require advanced skills to implement today.
Hardware will also likely play a role: secure enclave offerings being rolled out rapidly by the hyperscale cloud vendors and the associated confidential computing software platforms like Anjuna Security are making it easier to leverage these powerful hardware capabilities to secure AI systems and ensure privacy.
Operationalization and Edge Processing
While displaying information to humans is interesting and often useful, AI systems are the most valuable when they actually do something.
Systems that pair machine learning with optimization (e.g. routing, pricing), that can take action on their own using more traditional software automation – or that can produce content with generative models like GPT-3 or DALL-E – require lots of human expertise today. These systems often involve performing machine learning inference at the edge and also in central locations. Because of data gravity, pre-processing at the edge is often needed before sending data to central locations for training. Most software platforms are not designed for this kind of federated architecture (although SingleStore is doing good work in this space).
What Top AI Talent Wants
People with deep domain expertise in AI are often seeking to work in environments with academic-like cultures, ScaleUp economic upside, mission-oriented use cases, and access to large-scale compute infrastructures – as well as lots of training, inference and feedback data to fuel the learning systems they are building.
Some of the most substantive and fast-growing applied AI ScaleUps, such as Tractable, Overjet, Viz.ai, and Iterative Scopes, have been able to curate this type of environment, but it requires continuous focus, and it’s not easy.
Larger companies without patient investors willing to invest over long-term time horizons, or companies with equity that doesn’t have much potential for appreciation, are struggling even more with attracting and retaining AI talent.
For a more in-depth perspective of these and other topics, including how the adoption of AI is changing the relative defensibility of existing incumbent businesses versus emerging ScaleUps, check out my keynote session from ScaleUp:AI below.