Behind the Investment: Run:AI – Optimizing & Orchestrating AI and ML Resources
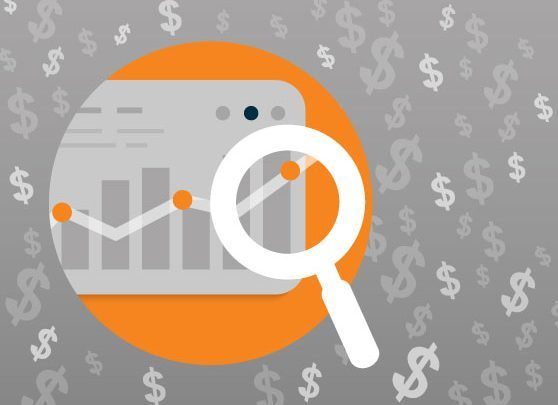
Data science, machine learning, and complex analytical workloads are increasingly becoming a core part of business processes. The amount of data that is created, ingested, and must be interpreted has drastically increased the need for computing power. There are a variety of different frameworks and hardware technologies one can use to run data-intensive workloads. Recently, graphics processing units (GPUs) and related specialty chips, such as Tensor Processing Units (TPUs), have emerged as the industry standard for many types of machine learning workloads.
GPUs are composed of thousands of specialized processing cores that can be used to speed up machine learning computations such as training (teaching a system how to make predictions using data) and inference (making predictions). Their highly parallelized architectures make them particularly well-suited for the calculations used to build, train, and deploy deep learning algorithms, such as those used for image recognition. Traditionally, GPU resources are allocated in a static way, often leaving expensive computer resources sitting idle, slowing down systems, and restricting data scientists’ productivity. Analysis shows that an average enterprise AI team may typically utilize as little as 25% of their GPU capacity.
That’s where Run:AI comes in. Run:AI’s platform enables businesses to virtualize their GPU and GPU-like resources. By more effectively sharing these resources, data science teams can minimize their compute infrastructure expense, while simultaneously ensuring that they have as much compute power as they need to bring their solutions to market. Much in the way VMware has served as the virtualization and management layer for VMs, so too does Run:AI have a similar opportunity for GPU and GPU-like hardware.
Customers have consistently conveyed the ease of use and demonstrable ROI of Run:AI, as they saw higher utilization rates from existing hardware and reduced the amount of redundant and idle jobs. Simply put, the platform’s ability to create fractional GPUs help redirect engineering efforts back to each customer’s core business.
Companies and their data science teams leverage Run:AI under the hood of their machine learning framework, toolkit, or MLOps platform, to handle the virtualization and management of their GPUs and GPU-like chipsets. In the public cloud these savings drop immediately to the bottom line and these performance improvements dramatically improve the responsiveness and accuracy of machine learning predictions. As data science teams have grown, these have become an increasingly important challenge to solve.
We had been following the progress of Run:AI since they were founded over 2 years ago. The extent of the solution’s sophistication came as no surprise once we met Omri, Ronen, and Meir – they are some of the brightest technologists we’ve had the pleasure to meet.
We are excited to be leading Run:AI's $30M Series B alongside existing investors, TLV Partners and S Capital. Looking ahead, Insight is excited to partner with Run:AI as they transition from a startup to a ScaleUp. With a clear value prop, growing market opportunity, and world-class team, we could not be more thrilled to be along for the ride!