The Rise of The Digital Worker to Scale Process and Systems at Will
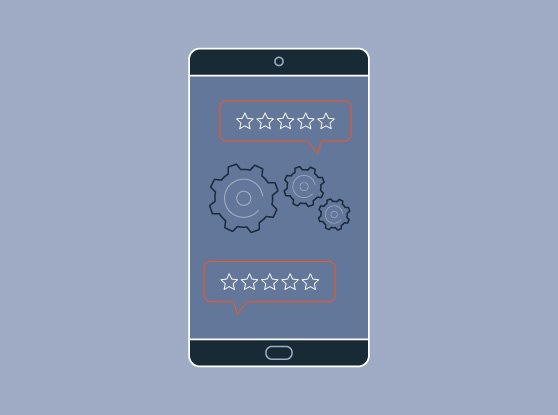
The magnitude of the global business problems we face today requires solutions at scale. Imagine in today’s world trying to solve a problem with a data set that fits on a 5 GB USB thumb drive. The number of customers, volume of data, and geo challenges SaaS companies face necessitate the ability to scale and adapt faster than ever before.
With widespread labor shortages and high demand for services, CTOs and CIOs ponder how to align human resources to support IT initiatives required to transform and scale their business. The new frontier they explore is a solution known as the “digital worker,” augmenting and extending the already stretched army of human workers. AI is not just an efficiency or productivity play. AI fundamentally improves the quality of business outcomes.
As defined by Forrester Research, digital worker-automation is “a combination of information architecture (IA) building blocks, such as conversational intelligence and robotic process automation (RPA), that works alongside employees. They understand human intent, respond to questions, and take action on the human's behalf, leaving humans with control, authority, and an enhanced experience.”
Today’s digital workers, like their human counterparts, have the ability to “sense,” “think” and “act.” The “act” capabilities — features like robotic process automation (RPA), business process workflows, APIs, and robotics — were the first introduced to the marketplace because they are the easiest to emulate. More recently, digital workers have been able to learn at a much faster rate than humans, helping organizations scale operations at will.
Digital workers can be used to speed up IT initiatives and allow organizations to have more breathing room for growth when human resources are lacking. In the long term, they can help companies scale by raising the ceiling of productivity IT companies will typically encounter. The application of the digital worker to deliver services in ways that were not previously humanly possible also represents future opportunities for those startups that are able to leverage AI.
AI to Scale
The process of scaling operations with the support of human and digital workers, in many ways, mirrors the “scale up and scale out” methodology of engineering departments: Businesses scale up by adding more human resources, and scale-out by augmenting their human resources with increasingly powerful digital resources that learn faster than humans.
The majority of the respondents in a recent KPMG survey of 950 business decision-makers said their organizations were adopting AI for business processes. Many of the respondents cited the pandemic as a key driver of AI adoption, with high adoption occurring among industries where a shortage of human workers could be adequately addressed by introducing digital workers. Industrial manufacturing, financial services, technology, and retail sectors all had adoption rates above 80%; life sciences had a rate of around 77%, and healthcare and government had adoption rates in the 60th percentile.
AI has the potential to fundamentally improve businesses, not just in terms of efficiency or productivity, but also in terms of quality of outcome and the range of services that can be offered. It’s important to note that AI doesn’t have to be restricted to a company’s core business to be successful. The ability of AI-driven digital workers to match many of the capabilities of a human worker in specialized tasks means applications like human resources and logistics management can be augmented with AI.
Since AI has entered the tech mainstream, companies in every sector of the economy — even those outside the tech industry — have found innovative ways to integrate machine learning (ML) into their core product. A 2020 survey found that 28% of manufacturing companies already use AI-assisted predictive maintenance, and more than half use data analytics to optimize resource allocation. Businesses that invest in AI for digital workers, even for the staff or support roles, can free up their human workers to deliver more services, at higher quality.
Real Business Outcomes
In the customer life cycle and journey, a lot of progress has been made through the application of AI in discoverability, acquisition and retention, engagement, efficiencies and ultimately scaling revenue. Business outcomes are better understood when tied to metrics like Annual Recurring Revenue (ARR), Year over Year (YoY) Bookings Growth, Year to Date (YTD) Sales Attainment, Customer Acquisition Cost (CAC), and Marketing Cost per New Opportunity.
A large number of companies today use some form of digital worker capable of “acting.” Workflow automation is a typical starting point. A popular form of automation is to replicate how humans act, enabling higher productivity or better-quality data. Companies like Taxjar, FloQast, M3ter, Smart Recruiters, Zenefits, Udemy, Qualtrics, Conga, Gainsight, Bynder, 6Sense, Monday, Workato, and many more rely on automated workflows to speed up business processes and empower human workers to focus on less repetitive tasks. An overwhelming 81% of tech leaders agree that workflow automation is a priority for their organization.
Process automation also has its challenges, however. If a flawed manual process is automated, it still remains flawed, and the negative impacts can be much higher because of the scale that automation enables. Automated processes are also poor at flexibility and handling evolving situations. This is where AI and the digital worker really shine, as the ability to “sense” and “think” empowers today’s AI engines to adapt to change and improve over time.
Ever-evolving sensing techniques help AI to collect and understand a diverse array of media and information types. Computer and machine vision allows machines to see the world around them so companies can automate visual inspection tasks that for a long time have required human workers.
Just as digital workers can see, they are now able to hear and listen. The distinction here is important — audio-detection technology predates AI by years (if not decades). But the ability to detect and identify sounds, or even transcribe them if they are spoken words, has opened a new world of automation for digital workers. The market for speech recognition is huge — a projected $29 billion by 2026.
Companies that are able to use speech recognition to automate tasks can position themselves in pole position to scale efficiently as the global economy starts to readjust to the impacts of the COVID-19 pandemic. A number of companies fall into the “thinking” and “sensing” categories of digital workers. This is by no means an exhaustive list, but it helps to illustrate the diversity of uses that AI can be put to:
- Writer leverages AI to help teams craft on-brand content. It provides snippets, checks for plagiarism and has custom writing style guides.
- 6Sense predicts when customer accounts hit buying-cycle stages and prompt execution. The platform engages buying teams with omnichannel and multi-touch campaigns.
- SmartRecruiters uses AI to find better hires with zero guesswork. The product screens new and existing resumés and surfaces matching candidates.
- DNSFilter has AI-powered security that protects from ransomware at the network level. It blocks known and zero-day ransomware domains to ensure employees do not inadvertently download malware.
- Explorium offers MLOps workflows for data enrichment for data security, feature generation, engineering, and other applications. This solution works at different levels of the MLOps toolchain: extraction, analysis, preparation, and ML-model iteration. Organizations can decide how much of their data science to outsource, from integrated-data management to full data science as a service (DSaaS) option.
Future Startups: ‘AI First’
This diversity of digital-worker AI applications gives today’s startups an unprecedented field of opportunity for success. Just as new companies 20 years ago had to think about being “digital-first” and ten years ago had to think about “cloud-first,” today’s companies must think about “AI-first.” Whether they currently use AI as part of their product offering or not to support digital workers, the future of the technology space, and of many sectors of the economy in general, will be driven by AI and digital workers. Companies have to be able to collect data at scale and build digital workers in a way that drives value for consumers. This also means being able to iterate on ML models, as they outlive their original usefulness for certain use cases and need to be retrained and realigned with new business goals.
Despite these challenges, today’s startups have an edge because of the existence of low-code or no-code platforms to support the digital worker framework. These platforms offer startups the ability to build automation, train pre-built ML models, and ship products without having to reinvent the wheel. This can be an asset for startups looking to provide proofs of concept or even working products while they build out their engineering and data science departments.
Bubble.io, Builder.ai, and Genesis are three emerging low-code platforms that allow companies to quickly and easily deploy apps. These platforms can also integrate with Amazon Personalize — AWS’s managed ML as a service offering — providing startups with the building blocks needed for creating a rich product that can be iterated and scaled up.
With such a large market for AI to empower digital workers, it can be difficult to identify the right moves. Companies must be able to spot emerging trends in the industry and stay ahead of the curve. The list of capabilities they must be able to provide include being able to:
1. Prioritize research-first rather than product-first
Tech startups have traditionally been extremely product-focused. AI startups generally take a fundamentally different approach, prioritizing research over product. This is largely due to how most AI scientists and engineers come from academia rather than industry, and many are new graduates. A 2020 Kaggle survey found that the majority of Kaggle data scientists had less than three years of experience.
Companies must be willing to invest in research and provide opportunities for AI scientists and engineers on staff to network and grow. This includes investments like attending conferences and publishing research journal papers that may not directly contribute to a marketable product.
2. Put data quality over model quality
Until recently, the standard AI pipeline has been to collect data, train a model, deploy to production and iterate over the algorithm until it reaches a level capable of filtering out noise and delivering accurate predictions. Comparatively, little attention has been devoted to the data and its labeling. This is changing, however, with the increasing focus being devoted to the quality of the data being gathered, data governance, and stewardship to ensure accurate and unbiased predictions.
As AI expands into new and exciting fields, scientists are discovering that no amount of model iteration or hyperparameter tuning will make up for sparse datasets with low-quality features. To successfully apply AI to novel disciplines for digital workers, startups must ensure that they have enough data to power their ML model and that the data is of sufficient quality.
3. Ensure security and privacy
Until now, security and privacy, and ML have often been at odds with one another. With different types of threat vectors associated with AI platforms, security becomes an even higher priority. Computer vision and decision-making can be tampered with by giving the system obscure edge cases, not experienced before. Injecting mislabeled data into the model training sets can also lead to misclassification and biased predictions.
Companies are realizing that amid a world rife with instability, consumers are starting to reject the convenience of ML if it means a complete loss of security and personal privacy. Differential privacy is an important trend changing the way companies do ML. An algorithm that fulfills data protection provides a mathematical guarantee of privacy — a guarantee that outputs of an AI algorithm cannot be traced back to any single member of the dataset, which often means a real-life individual. This is especially important as ML gains traction in fields like healthcare, where leakage of personal health information could have serious medical, financial, and legal consequences for patients, healthcare providers, and tech companies alike.
Defending ML models against ever-evolving security threats must be a priority for AI startups. Failure to keep AIs secure and user information private can erode investor and customer confidence, and threaten the public’s trust in a startup.
As you can see, the availability of digital workers and the AI market, in general, provide a tremendous opportunity for growth for companies seeking to scale to large enterprises.
We’re here to partner with you on your journey of scaling through digital workers — in addition to your existing army of humans. We can help you set up a sustainable approach and select scalable platforms to best meet your unique organizational needs. We also offer you the opportunity to learn from the successes and challenges of other portfolio companies and our growing partner community.